Author’s note: This is interestingly one of the most popular pieces on my blog, but it is one of my earliest and one that I’m least happy with. As my understanding of data science has evolved, I’ve come to see it as less nuanced than my later work. I initially approached the subject from a systems dynamics standpoint, while now I approach it with supervised statistical learning. While I still think the fundamental truths behind the post are valid, I’ve revised it to reflect my current understanding. The original blogpost is HERE.
Why is this the case? As physicians, we often seek a “silver bullet” – a single, effective solution with no side effects. The simplicity of Occam’s razor is alluring. One drug, one cure. The key fits the lock and opens the door. Penicillin cures Syphilis. But what if things are not that simple? What if treating some diseases is more like navigating a series of locked doors? A potential treatment may be blocked by the body’s own systems, such as drug elimination or homeostatic mechanisms. This complexity is often overlooked in experimental design, and it could be leading to a gap between in vitro success and in vivo applicability.
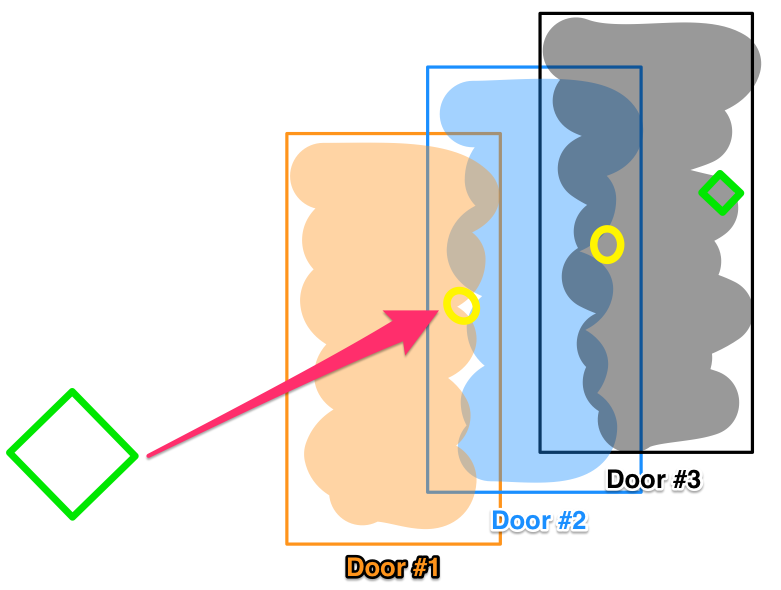
Let’s consider a locked door pathway, where opening Door #1 lets you pass through to Door #2, and so on sequentially to a final Door #3. Now imagine a drug that easily unlocks Door #3 , and effects a robust treatment, when tested in vitro. But in actual patients, systemic complexities block that drug from ever reaching Door #3. This is an oversimplification, of course. Biological systems are exponentially more complex than literal locked doors. But it illustrates how a drug’s efficacy can be foiled by confounding variables in vivo. Homeostatic mechanisms may identify the medicinal interloper as a threat. Like an adversarial immune response, the body’s regulatory processes counteract the treatment, as metabolism ramps up to accelerate its degradation before its target, or compensatory feedback loops are activated to blunt the response. Evolution has honed these defenses over eons – foiling our chemical intrusions.
With simple disorders, we’ve already plucked the low-hanging fruit. Prior generations found silver bullet treatments that directly and permanently relieved symptoms. The current shift from novel synthetic drugs to biologics may extend this opportunity and allow more treatments based on single drugs. But remaining diseases result from hugely complex and intersecting biological pathways, and silver bullets gave way to pattern recognition and statistical correlation – with mixed results.
When this post was originally written, a call to “revisit compounds that have initially discarded for reasons other than toxicity” was suggested. I’m happy to say that In Silico testing has become a reality in the interim. In no way I am claiming credit (although you never really know) but it was a likely outgrowth of application of statistical learning, tapping electronic health data. Repurposed drugs like phenoxybenzamine, entacapone and dimethyl fumarate have been the result.
However, a surfeit of data runs the risk of not only curve-fitting, but also increasing degrees of freedom and the number of comparisons runs the risk of discovering statistically significant, but spurious relationships. It is crucial to ensure that chance causality is excluded – hence the need for multi-dimensional statistical corrections like Holmes-Bonferroni and Bowmanini. Methods must be robust, and interpretations grounded in sound scientific and statistical reasoning.
Still, opportunity exists in mining troves of patient data to uncover statistical relationships and patterns between treatments, diseases, and outcomes. While randomized trials struggle to accommodate multiplying variables, big data analytics and longitudinal cross-validated EHR studies can add to the picture. They may reveal places where our existing tools, in new combinations, tested first in silico and only advanced to clinical trials when likely to succeed, can turn the tide on refractory illnesses inadequately treatable like vasospasm. This is in no way a solved problem – the answers we seek may already exist buried within the overwhelming noise of modern medicine’s myriad observations. But by listening to the data, we just may hear medicine’s deepest truths whispered through the cacophony.